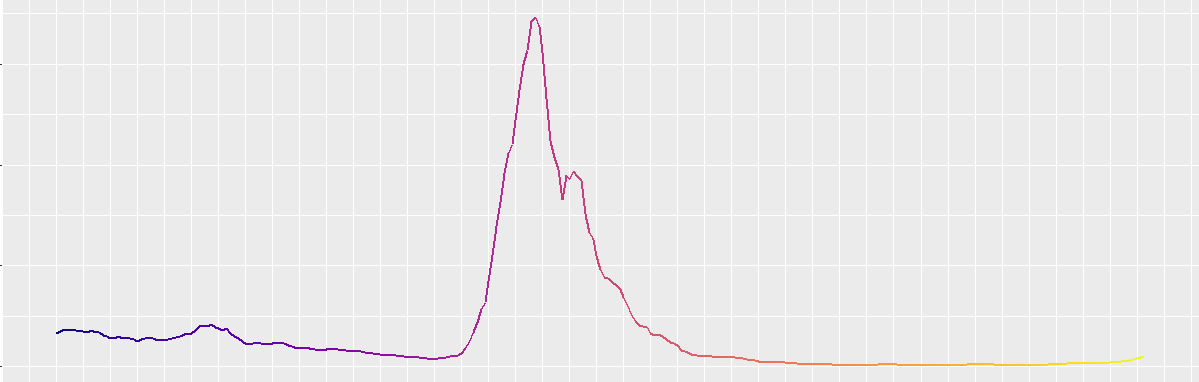
\[ \newcommand{\nRV}[2]{{#1}_1, {#1}_2, \ldots, {#1}_{#2}} \newcommand{\pnRV}[3]{{#1}_1^{#3}, {#1}_2^{#3}, \ldots, {#1}_{#2}^{#3}} \newcommand{\onRV}[2]{{#1}_{(1)} \le {#1}_{(2)} \le \ldots \le {#1}_{(#2)}} \newcommand{\RR}{\mathbb{R}} \newcommand{\Prob}[1]{\mathbb{P}\left({#1}\right)} \newcommand{\PP}{\mathcal{P}} \newcommand{\iidd}{\overset{\mathsf{iid}}{\sim}} \newcommand{\X}{\times} \newcommand{\EE}[1]{\mathbb{E}\left[{#1}\right]} \newcommand{\Var}[1]{\mathsf{Var}\left({#1}\right)} \newcommand{\Ber}[1]{\mathsf{Ber}\left({#1}\right)} \newcommand{\Geom}[1]{\mathsf{Geom}\left({#1}\right)} \newcommand{\Bin}[1]{\mathsf{Bin}\left({#1}\right)} \newcommand{\Poi}[1]{\mathsf{Pois}\left({#1}\right)} \newcommand{\Exp}[1]{\mathsf{Exp}\left({#1}\right)} \newcommand{\SD}[1]{\mathsf{SD}\left({#1}\right)} \newcommand{\sgn}[1]{\mathsf{sgn}} \newcommand{\dd}[1]{\operatorname{d}\!{#1}} \]
Chapter 3 Statistical Estimation
[Sherlock Holmes:] “The temptation to form premature theories upon insufficient data is the bane of our profession.”
— Arthur Conan Doyle (The Valley of Fear)
This chapter explores several key topics and techniques that play an important role in drawing meaningful conclusions from data. We begin by introducing the concept of an estimator, which is a statistical function used to estimate unknown parameters of a population based on sample data. We then look at the method of moments, a popular technique for estimating population parameters by equating sample moments with population moments. It then discusses maximum likelihood estimation, a powerful method for estimating parameters by maximising the likelihood function.
We also cover normality testing, which involves assessing whether data follow a normal distribution using graphical methods or statistical tests. Confidence intervals, which provide a range of plausible values for a population parameter along with a confidence level, are another important topic explored.
We also look at bivariate data, where two variables are analysed simultaneously. It includes concepts such as covariance and correlation, which quantify the relationship between two variables and help to understand their linear association.
Finally, we discuss the method of least squares, a widely used technique for estimating parameters in regression analysis. It focuses on minimizing the sum of squared residuals to obtain the best-fitting line or curve.
By covering these topics, this chapter provides readers with the tools and techniques necessary to estimate population parameters, assess data distributions, construct confidence intervals, analyse bivariate relationships, and perform regression analysis using the method of least squares. These methods are fundamental to statistical inference and play a crucial role in a wide range of fields, enabling researchers and practitioners to make informed decisions and draw meaningful insights from data.