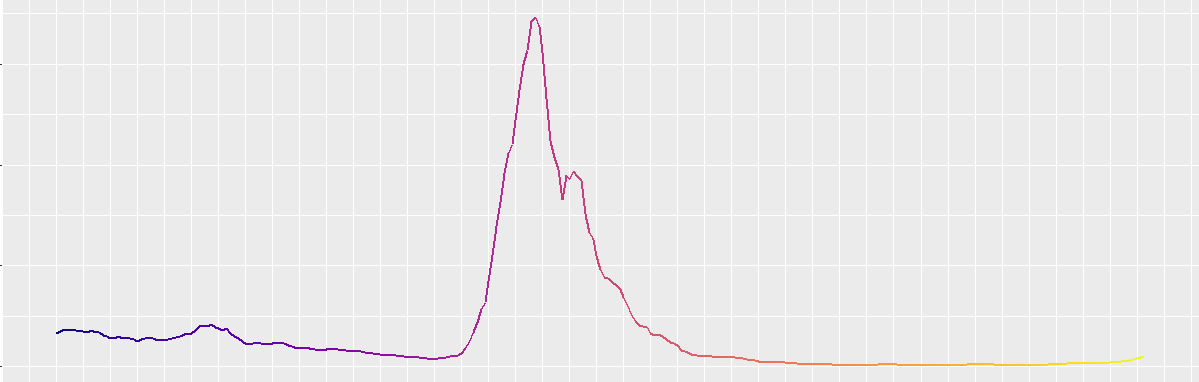
\[ \newcommand{\nRV}[2]{{#1}_1, {#1}_2, \ldots, {#1}_{#2}} \newcommand{\pnRV}[3]{{#1}_1^{#3}, {#1}_2^{#3}, \ldots, {#1}_{#2}^{#3}} \newcommand{\onRV}[2]{{#1}_{(1)} \le {#1}_{(2)} \le \ldots \le {#1}_{(#2)}} \newcommand{\RR}{\mathbb{R}} \newcommand{\Prob}[1]{\mathbb{P}\left({#1}\right)} \newcommand{\PP}{\mathcal{P}} \newcommand{\iidd}{\overset{\mathsf{iid}}{\sim}} \newcommand{\X}{\times} \newcommand{\EE}[1]{\mathbb{E}\left[{#1}\right]} \newcommand{\Var}[1]{\mathsf{Var}\left({#1}\right)} \newcommand{\Ber}[1]{\mathsf{Ber}\left({#1}\right)} \newcommand{\Geom}[1]{\mathsf{Geom}\left({#1}\right)} \newcommand{\Bin}[1]{\mathsf{Bin}\left({#1}\right)} \newcommand{\Poi}[1]{\mathsf{Pois}\left({#1}\right)} \newcommand{\Exp}[1]{\mathsf{Exp}\left({#1}\right)} \newcommand{\SD}[1]{\mathsf{SD}\left({#1}\right)} \newcommand{\sgn}[1]{\mathsf{sgn}} \newcommand{\dd}[1]{\operatorname{d}\!{#1}} \]
3.3 Maximum liklihood estimate
Definition 3.2 (Maximum likelihood estimator) The likelihood function for the sample \(X_1, X_2, \dots, X_n\) is, \[\begin{align*} L: \PP \X \RR^n \to \RR \textsf{ given by,} \\ L(p|{\bf X}) := \prod_{i=1}^n f(X_i|p) \end{align*}\] For a given \({\bf X} = (X_1, X_2, \dots, X_n)\) suppose \(\hat{p} \equiv \hat{p}({\bf X})\) is a point such that \(L(\hat{p}|{\bf X}) = \sup_{p \in \PP} L(p|{\bf X})\) ,i.e., \(L\) attains it’s maximum at \(\hat p\) as a function of \(p\). Then, \(\hat{p}\) is is called a maximum likelihood estimator of \(p\) (or abbreviated as MLE of \(p\)) given the sample \(X_1, X_2, \dots, X_n\)
Example 3.5 (Normal with one parameter) Let, \(X \sim N(p,1)\). Sampling \(X_1, X_2, \dots, X_n\) \(\rm iid\) from \(X\). Then the likelihood function \(L: \PP \X \R^n \to \R\) is given by,
\[\begin{align*}
\tag{3.9}
L(p|{\bf X})
&= \prod_{i=1}^n \frac{e^{-\frac{(X_i-p)^2}{2}}}{\sqrt{2\pi}} \\
&= \left( \frac{1}{\sqrt{2\pi}} \right)^n e^{-\sum_{i=1}^n \frac{(X_i-p)^2}{2}}
\end{align*}\]
To find MLE, treat \(X_1, X_2, \dots, X_n\) as fixed and maximize \(L\) as a function of \(p\)
Observe that, \(\exp(-x)\) is a strictly decreasing function of \(x\).
Figure 3.1: \(\exp(-x)\)
and, \(\left( \frac{1}{\sqrt{2\pi}} \right)^n\) is constant w.r.t. \(p\). So, maximizing \(L(p|{\bf X})\) is equivalent to minimizing \(g : \RR \to \R\) with \(g(p) = \sum_{i=1}^n (X_i-p)^2\)
Method 1 (Using inequality)
\[\begin{align*}
g(p)
&= \sum_{i=1}^n (X_i-p)^2 \\
&= \sum_{i=1}^n \left(X_i - \overline{X} +\overline{X} - p\right)^2 \\
&= \sum_{i=1}^n \left(X_i - \overline{X}\right)^2 + n(\overline{X} - p)^2
\end{align*}\]
Observe that, \(g(p) \geq \sum_{i=1}^n \left(X_i-\overline{X}\right)^2 \ \forall p\in\R\) and \(g(\overline{X}) = \sum_{i=1}^n \left(X_i-\overline{X}\right)^2\)
Thus, MLE of \(p\) given \(X_1, X_2, \dots, X_n\) is \(\hat{p} = \overline{X}\)
Method 2 (Using calculus)
\[\begin{align*} g'(p) &= -2\sum_{i=1}^n \left(X_i-p\right) \\ \textsf{ and, } g''(p) &= 2 > 0 \end{align*}\] Now, \[\begin{align*} g'(p) = 0 \implies \sum_{i=1}^n \left(X_i-p\right) = 0 \implies p = \overline{X} \textsf{ and, } g''(\overline{X}) > 0 \end{align*}\] \(\therefore p=\overline{X}\) is a local minimum (global too) (See Exercise 3.4) of \(g\). Hence, \(\hat{p}=\overline{X}\) is the MLE of \(p\) given \(X_1, X_2, \dots, X_n\)
Exercises
Exercise 3.4 (Form Example 3.5) Show the following:
- Show using elementary algebra, \[\sum_{i=1}^n \left(X_i - p\right)^2 = \sum_{i=1}^n \left(X_i-\overline{X}\right)^2 + n(\overline{X} - p)^2\]
- Show that \(p=\overline{X}\) is the global minimum of \(g\).
Exercise 3.5 (Raleigh Distribution) Gobarkanth collects \(X_1, X_2, \dots, X_n\) of \(\rm iid\) measurements of radiation from Gobar Gas plant of canteen. He assumes that the observations follow \(\alpha\) Rayleigh distribution with parameter \(\alpha\), with pdf given by, \[\begin{equation*} f(x) = \begin{cases} \alpha x \exp{\left( -\frac{1}{2}\alpha x^2 \right)} & \textsf{ if } x \geq 0 \\ 0 & \textsf{ otherwise } \end{cases} \end{equation*}\] Find the maximum likelihood estimate for \(\alpha\), providing appropriate justifcation.
Exercise 3.6 (capture-recapture and hypergeometric distribution) Biologists use a technique called capture-recapture to estimate the size of the population of a species that cannot be directly counted. The following exercise illustrates the role a hypergeometric distribution plays in such an estimate.
Suppose there is a species of unknown population size \(N\). Suppose fifty members of the species are selected and given an identifying mark. Sometime later a sample of size twenty is taken from the population and it is found that four of the twenty were previously marked.
- \(N\) be the number of population in the wild. Write down the likelihood function for \(N\) given the above data.
- Plot the likelihood function for \(N\).
- Use the
optimize()
function in R to find the maximum likelihood estimate for \(N\). - Can you compute the MLE for \(N\) using calculus?
The basic idea behind mark-recapture is that since the sample showed \(\frac{4}{20} = 20\%\) marked members, that should also be a good estimate for the fraction of marked members of the species as a whole. However, for the whole species that fraction is \(\frac{50}{N}\) which provides a population estimate of \(N \approx 250\)
Exercise 3.7 (Head Tail) A coin is flipped \(100\) times and \(55\) heads occurred. Assume that the coin has probability of heads being \(p\).
- Write an R-function that will compute the value of the likelihood function for any value of \(p\).
- Plot the likelihood function for \(p \in (0,1)\).
- Use the
optimize()
function in R to find the maximum likelihood estimate for \(p\). - Compute the MLE for \(p\) using calculus and see how close is to the answer in the previous step.
- Do the above steps if the number of observed heads was \(30\), and \(70\).
Exercise 3.8 (Binomial Distribution) Suppose we have n samples \(X_1, X_2, \dots, X_n\) from \(\Bin{N,p}\). We are told the value of \(M = \max{\left\{ X_1, X_2, \dots, X_n \right\}}\).
- Find the Probability mass function of \(M\).
- Write an R-function that will compute the value of the likelihood function \(L(p | M, N, n)\) for any value of \(p\).
- Suppose \(M = 30, N = 50, n = 10\).
- Plot the likelihood function for \(p \in (0,1)\).
- Use the
optimize()
function in R to find the maximum likelihood estimate for \(p\). - Can you compute the MLE for \(p\) using calculus?
- Do the previous step if \(M\) is now \(20\), or \(40\).
Exercise 3.9 (Diploid Genotype) Suppose that a particular gene occurs as one of two alleles (\(A\) and \(a\)), where allele \(A\) has frequency \(\theta\) in the population. That is, a random copy of the gene is \(A\) with probability \(\theta\) and \(a\) with probability \((1 - \theta)\). Since a diploid genotype consists of two genes, the probability of each genotype is given by:
Genotype | \(AA\) | \(Aa\) | \(aa\) |
Probability | \(\theta^2\) | \(2\theta(1-\theta)\) | \((1-\theta)^2\) |
Suppose we test a random sample of the population and find that \(k\) are \(AA\), \(l\) are \(Aa\), and \(m\) are \(aa\).
- Write an R-function that will compute the value of the likelihood function for any value of \(\theta\) given \(k, l, m\).
- Suppose \(k = 10, l = 30, m = 20\).
- Plot the likelihood function for \(\theta \in (0,1)\).
- Use the
optimize()
function in R to find the maximum likelihood estimate for \(\theta\). - Compute the MLE for \(\theta\) using calculus and see how close is to the answer in the previous step.
- Do the previous step if the \(k = l = m = 30\).