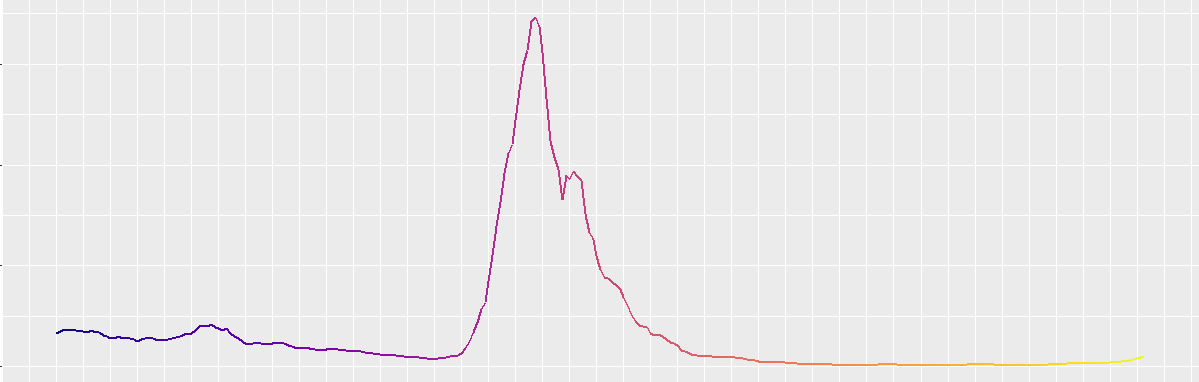
\[ \newcommand{\nRV}[2]{{#1}_1, {#1}_2, \ldots, {#1}_{#2}} \newcommand{\pnRV}[3]{{#1}_1^{#3}, {#1}_2^{#3}, \ldots, {#1}_{#2}^{#3}} \newcommand{\onRV}[2]{{#1}_{(1)} \le {#1}_{(2)} \le \ldots \le {#1}_{(#2)}} \newcommand{\RR}{\mathbb{R}} \newcommand{\Prob}[1]{\mathbb{P}\left({#1}\right)} \newcommand{\PP}{\mathcal{P}} \newcommand{\iidd}{\overset{\mathsf{iid}}{\sim}} \newcommand{\X}{\times} \newcommand{\EE}[1]{\mathbb{E}\left[{#1}\right]} \newcommand{\Var}[1]{\mathsf{Var}\left({#1}\right)} \newcommand{\Ber}[1]{\mathsf{Ber}\left({#1}\right)} \newcommand{\Geom}[1]{\mathsf{Geom}\left({#1}\right)} \newcommand{\Bin}[1]{\mathsf{Bin}\left({#1}\right)} \newcommand{\Poi}[1]{\mathsf{Pois}\left({#1}\right)} \newcommand{\Exp}[1]{\mathsf{Exp}\left({#1}\right)} \newcommand{\SD}[1]{\mathsf{SD}\left({#1}\right)} \newcommand{\sgn}[1]{\mathsf{sgn}} \newcommand{\dd}[1]{\operatorname{d}\!{#1}} \]
Chapter 4 Hypothesis Testing
“It’s easy to lie with statistics. It’s hard to tell the truth without statistics”
— Andrejs Dunkels
The concept of hypothesis testing provides a framework for comparing an observed quantity from a sample (such as \(\overline{X}\)) with an expected result based on an assumption about the distribution of \(X\). There are several types of hypothesis tests, and in this discussion we will focus on four commonly used tests: the \(Z\) test, the \(t\) test, the \(F\) test, and the \(\chi^2\) test. This is not an exhaustive list, but it will provide an overview of these particular forms of hypothesis testing.
In hypothesis testing, the “null hypothesis” (usually denoted as \(H_0\) or \(H\)) represents a specific assumption about the distribution of \(X\). This null hypothesis is then compared with an “alternative hypothesis” (usually denoted as \(H_A\) or \(K\)), which proposes a specific departure from the null assumption. The aim is to assess whether the observed sample data is significantly different from what would be expected if the null hypothesis were true.
To perform the hypothesis test, a calculation is performed based on the differences between the sample data and the expected outcome under the null hypothesis. This calculation yields a quantity known as the “\(p-\)value”, which indicates the probability of observing a sample as extreme as the one actually obtained, assuming the null hypothesis is true. The interpretation of the \(p-\)value depends on the specific test being conducted and the assumptions of the null hypothesis.
A small \(p-\)value indicates that the sample is highly unlikely under the null hypothesis, casting doubt on its validity. Conversely, a large \(p-\) value indicates that the sample is quite consistent with the assumptions of the null hypothesis. By comparing the \(p-\) value with a predetermined significance level (usually denoted by \(\alpha\)), we can make decisions about rejecting or accepting the null hypothesis.
Note that the process of comparing observation with expectation varies depending on the type of test performed and the specific assumptions underlying the null hypothesis. Hypothesis testing is a powerful tool for making statistical inferences and drawing conclusions based on sample data and pre-defined hypotheses.